Data Science Association Rule Learning use case.
Python programming language and its libraries combined together and R language in addition form the powerful tools for solving Association Rule Learning tasks.
Association rule learning is a rule-based machine learning ML method for detecting possible relations between variables in large datasets. It's purpose is to identify powerful rules discovered in datasets using some measures of interestingness.
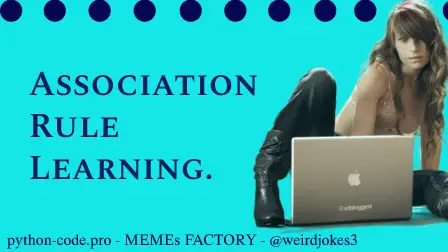
Python Knowledge Base: Make coding great again.
- Updated:
2025-07-12 by Andrey BRATUS, Senior Data Analyst.
Apriori model.
Eclat model.
The idea behind Association Rule Learning is quite simple: given a set of transactions, find rules that will predict the occurrences of an item based on the occurrences of other items in the transactions. In other words, discovering relations between variables in the data and perdict possible users' behaviour.
Apriori in Python.
#Importing the libraries
!pip install apyori
import numpy as np
import matplotlib.pyplot as plt
import pandas as pd
#Importing the dataset and Data Preprocessing
dataset = pd.read_csv('my_dataset.csv', header = None)
transactions = []
for i in range(0, 7501):
transactions.append([str(dataset.values[i,j]) for j in range(0, 20)])
#Training the Apriori model on the dataset
from apyori import apriori
rules = apriori(transactions = transactions, min_support = 0.003, min_confidence = 0.2, min_lift = 3, min_length = 2, max_length = 2)
#Visualising the results
results = list(rules)
def inspect(results):
lhs = [tuple(result[2][0][0])[0] for result in results]
rhs = [tuple(result[2][0][1])[0] for result in results]
supports = [result[1] for result in results]
confidences = [result[2][0][2] for result in results]
lifts = [result[2][0][3] for result in results]
return list(zip(lhs, rhs, supports, confidences, lifts))
resultsinDataFrame = pd.DataFrame(inspect(results), columns = ['Left Hand Side', 'Right Hand Side', 'Support', 'Confidence', 'Lift'])
resultsinDataFrame.nlargest(n = 10, columns = 'Lift')
Apriori in R
#Importing the dataset and Data Preprocessing
# install.packages('arules')
library(arules)
dataset = read.csv('my_dataset.csv', header = FALSE)
dataset = read.transactions('my_dataset.csv', sep = ',', rm.duplicates = TRUE)
summary(dataset)
itemFrequencyPlot(dataset, topN = 10)
#Training Apriori on the dataset
rules = apriori(data = dataset, parameter = list(support = 0.003, confidence = 0.2))
# Visualising the results
inspect(sort(rules, by = 'lift')[1:10])
Eclat in Python.
#Importing the libraries
!pip install apyori
import numpy as np
import matplotlib.pyplot as plt
import pandas as pd
#Importing the dataset and Data Preprocessing
dataset = pd.read_csv('my_dataset.csv', header = None)
transactions = []
for i in range(0, 7501):
transactions.append([str(dataset.values[i,j]) for j in range(0, 20)])
#Training the Eclat model on the dataset
from apyori import apriori
rules = apriori(transactions = transactions, min_support = 0.003, min_confidence = 0.2, min_lift = 3, min_length = 2, max_length = 2)
#Visualising the results
results = list(rules)
def inspect(results):
lhs = [tuple(result[2][0][0])[0] for result in results]
rhs = [tuple(result[2][0][1])[0] for result in results]
supports = [result[1] for result in results]
return list(zip(lhs, rhs, supports))
resultsinDataFrame = pd.DataFrame(inspect(results), columns = ['Product 1', 'Product 2', 'Support'])
resultsinDataFrame.nlargest(n = 10, columns = 'Support')
Eclat in R.
#Importing the dataset and Data Preprocessing
# install.packages('arules')
library(arules)
dataset = read.csv('my_dataset.csv', header = FALSE)
dataset = read.transactions('my_dataset.csv', sep = ',', rm.duplicates = TRUE)
summary(dataset)
itemFrequencyPlot(dataset, topN = 10)
#Training Eclat on the dataset
rules = eclat(data = dataset, parameter = list(support = 0.003, minlen = 2))
# Visualising the results
inspect(sort(rules, by = 'support')[1:10])